Make informed design decisions early on by quantifying millions of architectures virtually
Architecture analysis, whether it is a powertrain architecture or a cooling system architecture, ensures that the system architectures are aligned with desired requirements and that all the possibilities are thoroughly explored. It is an essential aspect of Model-Based Systems Engineering, (MBSE), an approach where all requirements are captured and converted into a model showing the relationship between function and requirements. In this article, we will explore an architecture analysis technique with generative engineering within the realm of MBSE. We will also showcase a case study of cooling architecture analysis for electric vehicles (EVs) to demonstrate the practical application of these techniques.
The current state of the art in automotive architecture selection often involves a time-consuming and iterative process of evaluating and refining concepts based on past experiences and expert judgment. This process can be subjective, prone to biases, and limited by the knowledge and experiences of the individuals involved. It may also overlook certain trade-offs and system interactions that can significantly impact the overall performance and efficiency of the automotive architecture. As automotive systems become more complex, interconnected, and technologically advanced, there is a growing need for a more systematic and comprehensive approach to concept selection that goes beyond the limitations of the existing state of the art.
Generating ideas faster and bringing products to market more quickly
Generative engineering is an iterative design and engineering process that uses AI to generate outputs based on a set of criteria. It allows engineers to quickly iterate and select the best design options. It is particularly valuable for solving difficult problems, such as early architectural design explorations.
Generative Engineering in architecture exploration is complemented by trade-off simulation & analysis, which quantifies the benefits and drawbacks of architectural alternatives, leading to more informed design decisions. By creating virtual models and subjecting them to simulated scenarios, engineers can assess system performance and other key attributes. Simulations enable the evaluation of architectural alternatives under various conditions, providing a comprehensive understanding of system behavior.
Simcenter Studio software from Siemens offers generative engineering solutions that help manufacturers make a holistic assessment of alternative system architectures. A team of experts from multiple disciplines within your organization can work together to incorporate a broad range of requirements and tie them to simulation or test, to define a system model. From that central model, the software automatically explores every possible alternative system architecture, intelligently ranking and promoting them to ensure you make your selection from the best options available.
Generative engineering involves systematically generating and evaluating a wide range of architectural alternatives within predefined constraints. This approach encourages creativity and innovation by uncovering novel configurations that may not have been considered using traditional methods. Engineers can manually explore the design space or leverage automated algorithms to discover optimal designs.
For more information on how AI-driven MBSE can help to find a truly innovative direction at the very earliest stages of your design cycle, read this blog post: MBSE driven by AI – shake that design fixation!
Exploring alternative architecture analysis of cooling systems for an electric vehicle
Efficient cooling systems are vital to maintain optimal performance and prevent damage to sensitive components.
The vehicle architecture analysis of internal combustion engines often focuses on optimizing a single cooling objective, such as maintaining a specific temperature range for the engine. However, for an electrified vehicle, there are multiple components that need to be maintained at different temperatures. The cooling system now needs to serve many targets and objectives. The engine still needs to be maintained at 95 C° but the lithium-ion battery is at around 35 C° and the electric motor somewhere in the middle, around 65 C°. Embracing multi-objective optimization techniques allows engineers to consider additional objectives, such as minimizing energy consumption and reducing system complexity.
Using a model-based approach, engineers can create a virtual representation of the electric vehicle and its cooling system in a system simulation tool such as Simcenter Amesim. This model includes parameters such as ambient temperature, battery temperature, weight, and cost. By subjecting the model to various simulated driving scenarios, your engineers can evaluate different cooling architectures and assess their performance under different operating conditions.
Automatically evaluating EV cooling design alternatives
At its core, generative engineering starts by capturing the requirements and constraints of a specific problem or system. These requirements could include factors like performance goals, safety regulations, material limitations, or cost targets. By inputting these parameters into the generative engineering framework, engineers create a design space that can be systematically explored.
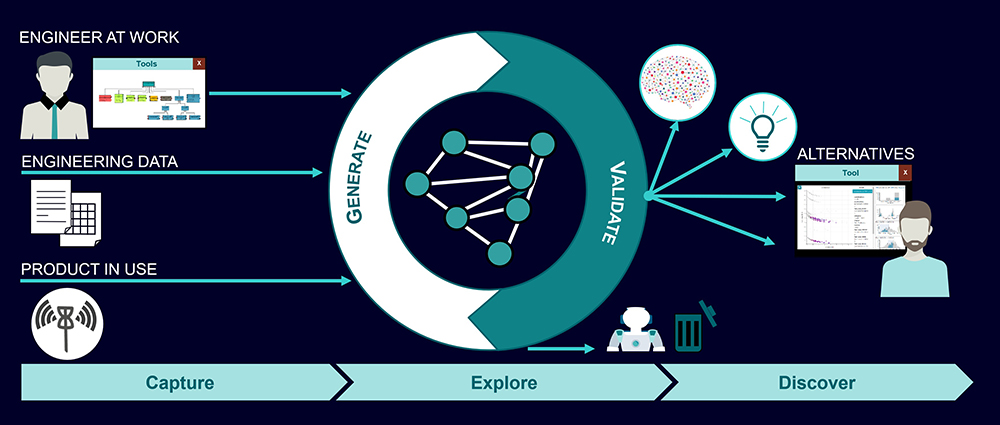
Using advanced algorithms, generative engineering generates a wide array of design alternatives that satisfy the specified requirements. These designs are often innovative and unconventional, stretching beyond the boundaries of what human designers might conceive. By exploring this vast design space, engineers can discover novel solutions that were previously unknown or unexplored.
Simcenter Studio’s use of AI in generative engineering allows Siemens to design the thermal cooling system architecture for the demonstrator electric vehicle, Simrod, which was optimized for power consumption, cost, and weight. This methodology leverages advanced algorithms and computational models to explore a vast design space and identify optimal solutions.
With generative engineering we created numerous designs that operated within specified temperature limits while delivering efficient performance. By considering three different temperature scenarios and two drive cycles, this process enables comprehensive evaluation and robustness analysis.
Through generative engineering, various design parameters such as heat exchanger configurations, coolant flow rates, and fan placements are systematically explored and iterated upon. The algorithms intelligently generate and evaluate numerous design alternatives, optimizing for power consumption, cost, and weight simultaneously.
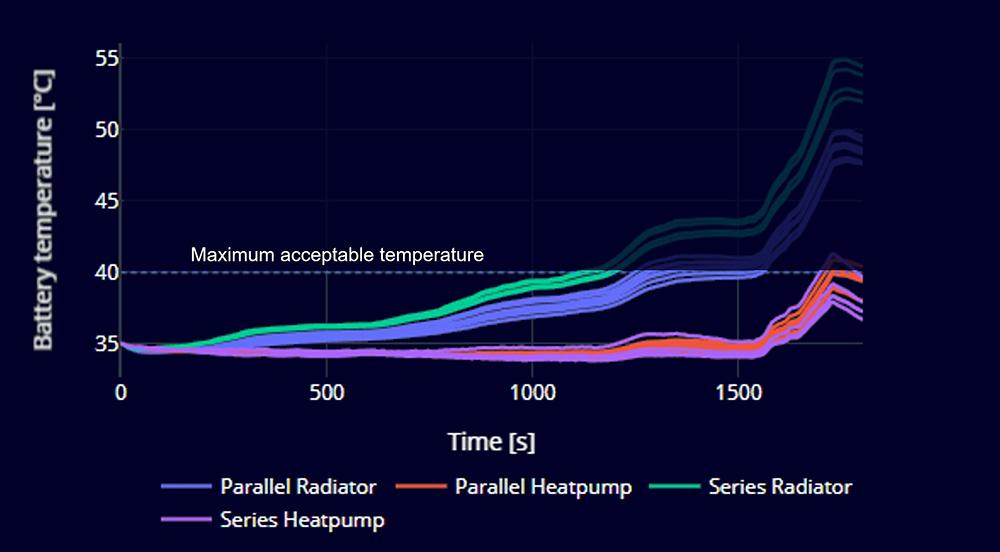
The resulting thermal cooling system architecture for the Simrod was able to achieve a fine balance between thermal performance and resource efficiency. It offers enhanced cooling capabilities, ensuring temperature control under different conditions, while also minimizing power usage, reducing costs, and maintaining a lightweight profile. Generative engineering allowed our engineers to efficiently and effectively design an advanced thermal cooling system that met diverse requirements and outperformed traditional design approaches.
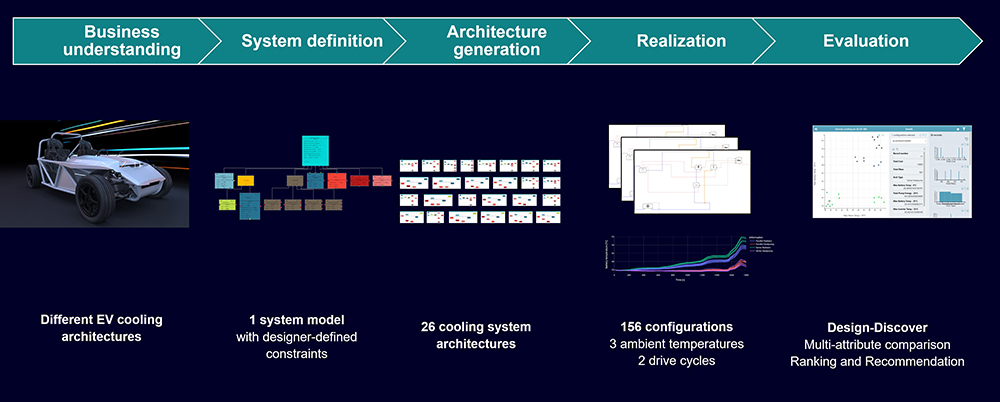
How to take maximum advantage of AI-driven generative engineering
Generative AI is an incredible technology, but it’s still just a technology. To take maximum advantage of it, companies need to rewire so they can rapidly develop solutions, improve their customer experience, accelerate innovation, and reduce costs.
In case you experience project backlogs or need simulation capability , you can partner with Simcenter Engineering and Consulting experts to meet your unique needs. The team brings critical expertise to your process with proven product design services that address your most critical development challenges.
In conclusion, alternative architecture analysis techniques offer valuable enhancements to traditional methods of feasibility analysis and architecture definition stage in Model-based System Engineering. Embracing generative engineering and system simulation can significantly improve the efficiency and effectiveness of the architecture analysis process. By incorporating these approaches into the Model-Based Systems Engineering framework, engineers can optimize system performance, make informed design decisions, and ultimately create robust systems that successfully fulfill multiple objectives much early in the development process. These alternative techniques foster innovation and elevate the overall quality of system design.
where can i buy lasuna – himcolin pills order himcolin generic
besifloxacin over the counter – carbocisteine oral purchase sildamax online cheap
gabapentin 600mg over the counter – ibuprofen brand azulfidine 500mg pills
benemid price – brand probenecid purchase tegretol online cheap
celecoxib 100mg price – flavoxate medication indocin 50mg oral
buy colospa no prescription – colospa 135 mg for sale pletal 100mg for sale
diclofenac 50mg ca – order aspirin 75 mg sale purchase aspirin generic
purchase rumalaya without prescription – buy rumalaya online buy amitriptyline online
pyridostigmine 60mg us – buy pyridostigmine 60 mg without prescription azathioprine 50mg for sale
purchase mobic generic – rizatriptan over the counter toradol cost
order generic cyproheptadine 4 mg – buy tizanidine pills for sale tizanidine cheap
artane cost – purchase emulgel online buy voltaren gel online
order cefdinir 300mg generic – brand clindamycin generic clindamycin
accutane 10mg tablet – order deltasone 40mg without prescription deltasone online order
buy acticin generic – benzoyl peroxide without prescription tretinoin brand
buy generic betamethasone online – betamethasone 20 gm drug purchase benoquin sale
buy flagyl 400mg for sale – flagyl order online cenforce 50mg uk
order amoxiclav for sale – augmentin online purchase levoxyl generic
cleocin 150mg for sale – order indomethacin 75mg capsule indomethacin 50mg usa
order losartan for sale – order cozaar online keflex price
crotamiton for sale online – aczone ca aczone online order
modafinil 100mg ca – buy provigil without a prescription brand melatonin 3mg
bupropion us – cheap shuddha guggulu buy shuddha guggulu pill
capecitabine uk – buy xeloda online cheap how to buy danazol
order prometrium online cheap – prometrium uk buy clomiphene no prescription
alendronate us – order pilex without prescription provera 5mg brand
yasmin cheap – buy letrozole 2.5 mg sale anastrozole order
dostinex 0.25mg tablet – cabgolin price alesse price
г‚·гѓ«гѓ‡гѓЉгѓ•г‚Јгѓ«йЂљиІ© – г‚їгѓЂгѓ©гѓ•г‚Јгѓ« жµ·е¤–йЂљиІ© г‚·г‚ўгѓЄг‚№йЂљиІ©гЃЉгЃ™гЃ™г‚Ѓ
eriacta alice – forzest countenance forzest harmony
valif pills peaceful – sinemet 20mg uk sinemet 20mg drug
provigil generic – provigil 100mg pills buy lamivudine cheap
promethazine 25mg us – order ciplox 500mg without prescription order lincomycin 500 mg pills
ivermectin 12 mg over the counter – how to buy candesartan tegretol 400mg sale
buy prednisone 20mg sale – starlix 120mg pill buy generic capoten online
order deltasone 20mg pills – order captopril 25 mg captopril 25mg brand
buy accutane 40mg online – zyvox 600mg uk buy linezolid generic
amoxil cost – diovan where to buy buy ipratropium paypal